SAP & Oracle partner and support companies
SAP & Oracle consulting Expertise
Explore MoreWe would love to tell you about us!
15 Long Years Of SAP & Oracle Excellence With Quality At It’s Best!
We are a team of passionately dedicated professionals who came together in the year 2016 and formed Ayoshya technology. In the time when the entire world is fighting the global epidemic and businesses are striving to survive, we can help your business sail smoothly by providing efficient and cost-effective SAP Services. We can help you gain momentum in this digital world.We can deliver quality-oriented and time-driven SAP functional and technical consulting to different business horizons such as Information Technology, Manufacturing, Construction, Media & Hospitality, Financial Services, and many others.
From SAP implementation to SAP migration, get everything that you need in one place. If you want to know how we can add value to your business, get in touch with our experts today!
Learn MoreRedefine your customer experience with our SAP & Oracle expertise
SAP & ORACLE CONSULTING SERVICES
- Ayoshya SAP S/4 HANA Services
- SAP Template Design and Implementation /Rollout
- SAP GST Readiness and Migration
SAP & ORACLE MANAGED SERVICES
- SAP & Oracle Administration and Support
- SAP & Oracle Business Process Optimization
- Offshore ABAP Development Services
DEVELOPMENT SERVICES
- Offshore ABAP Development Services
- SAP Intelligent Robotic Processes Automation (RPA)
- Customized ERP Solutions Services
The Only Place Where You’ll Get The Perfect Solution For All Your EPR Solutions.
Learn MoreOur Contributions
Industries We Serve!
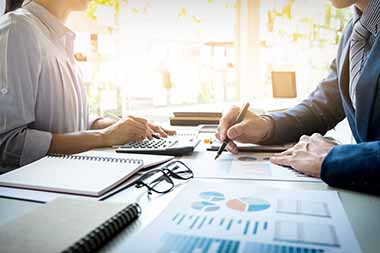
SAP & ORACLE Consulting Services
- SAP & Oracle Implementation / Rollout
- SAP & Oracle Re-implementation
- SAP Upgrade / Migration
- SAP & Oracle Audit
- SAP & Oracle Training
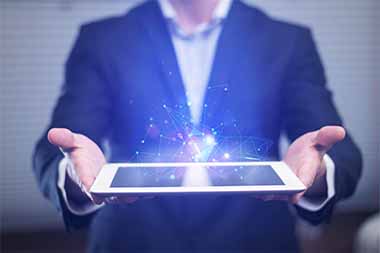
SAP & Oracle Managed Services
- SAP & Oracle Application Support
- Offshore ABAP Development Services
- SAP & Oracle Value Added Solutions
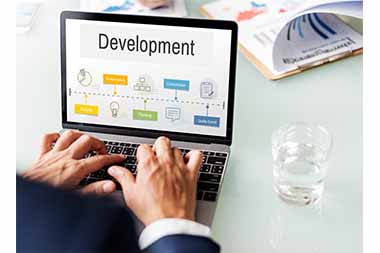
Development Services
- Offshore ABAP Development Services
- SAP Intelligent Robotic Processes Automation (RPA)
- Customized ERP Solutions Services