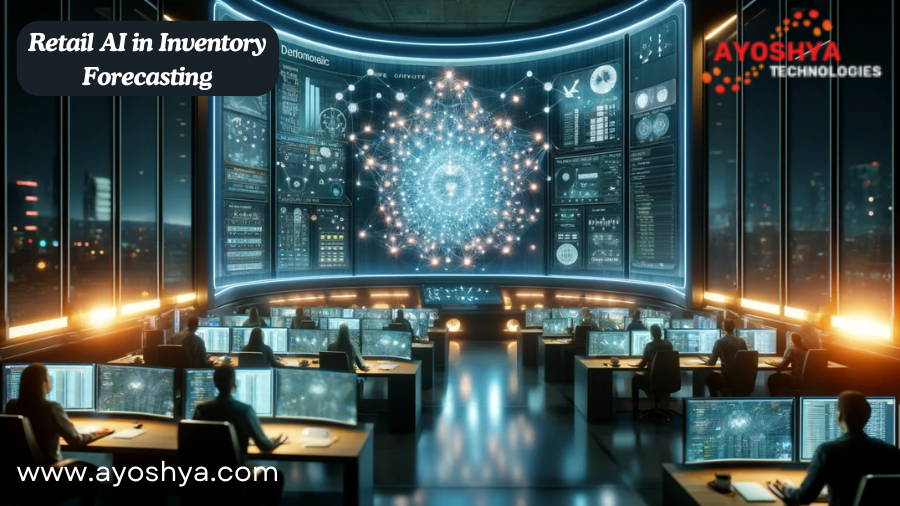
Dramatically improve forecast accuracy and optimize stock levels with Retail AI. Reduce stockouts, eliminate overstocking, and boost profits… Learn more about AI inventory forecasting for retailers!**
Have you ever stared down a shelf completely empty of your favorite product, frustration bubbling as you walk away empty-handed? Or maybe you’ve witnessed aisles overflowing with outdated stock, a sinking feeling knowing those items will likely end up discounted or worse, thrown away. These are the harsh realities of traditional inventory management, a constant battle against stockouts and overstocking. But fear not, retail warriors! A revolution is brewing, powered by the magic of Artificial Intelligence (AI). Retail AI in Inventory Management Forecasting is here to transform the way you manage your stock, ensuring you have the right products, in the right quantities, at the right time. Imagine a world where you can predict demand with pinpoint accuracy, optimize inventory levels, and say goodbye to lost sales and wasted resources. This is the future of retail, and it’s closer than you think.
2. How Does Retail AI Work for Inventory Forecasting?
Traditional inventory forecasting methods often rely on static models that consider historical sales data but fail to account for the dynamic nature of the retail landscape. Human error can also significantly impact the accuracy of these forecasts. Retail AI offers a revolutionary approach that leverages the power of machine learning to analyze vast amounts of data and identify complex patterns that influence demand. This section delves into the inner workings of Retail AI and how it transforms inventory forecasting.
Core Concepts of AI-powered Forecasting
At the heart of Retail AI forecasting lies machine learning, a branch of artificial intelligence that allows computers to “learn” from data without explicit programming. AI models are trained on historical sales data, encompassing factors like product popularity, seasonality, and promotional activity. Over time, these models become adept at recognizing patterns and relationships within the data. This enables them to make increasingly accurate predictions about future demand for specific products.
Machine learning algorithms used in Retail AI can be broadly categorized into two main types: regression analysis and time series forecasting. Regression analysis identifies the statistical relationships between sales data and various influencing factors. For instance, an AI model might identify a strong correlation between a product’s price and its sales volume. Time series forecasting algorithms analyze historical sales data patterns over time, such as seasonal trends and cyclical fluctuations. By combining these techniques, Retail AI can create highly accurate forecasts that account for both short-term and long-term trends in demand.
Data Used by AI Models
To achieve optimal forecasting accuracy, Retail AI models require a rich and diverse dataset. The foundation lies in historical sales data, which provides insights into past demand patterns for individual products and product categories. However, AI goes beyond just sales figures. Modern forecasting models can incorporate a wide range of external data sources that can significantly influence demand.
These external data sources can be broadly categorized into three main areas:
- Market Trends: Including data on economic indicators, consumer confidence levels, and competitor activity. By analyzing these trends, AI models can anticipate shifts in consumer behavior and adjust forecasts accordingly.
- Weather Patterns: For certain product categories, weather can significantly impact demand. For example, AI models can incorporate weather forecasts to predict a surge in demand for sunscreen or raincoats based on anticipated weather conditions.
- Social Media Sentiment: Social media provides a valuable real-time pulse on consumer sentiment. AI can analyze social media conversations to identify emerging trends, product buzz, and potential brand crises. This information can be used to proactively adjust inventory levels for products experiencing sudden popularity or avoid overstocking items facing negative social media sentiment.
Implementation Considerations for Retail AI
Successfully implementing Retail AI for inventory forecasting requires careful planning and consideration of several key factors. Here, we’ll delve deeper into each of these aspects to ensure a smooth and successful transition for your business.
Data Quality and Integration
The success of any AI model hinges on the quality and accuracy of the data it is trained on. “Garbage in, garbage out” applies very much to AI systems. For retail AI in inventory forecasting, this translates to ensuring your data is clean, complete, and up-to-date.
- Data Cleaning and Standardization:
Retailers often have data scattered across various systems like sales platforms, point-of-sale systems, and customer relationship management (CRM) software. The first step is to consolidate this data into a centralized repository. During this process, identify and rectify any inconsistencies, missing values, or duplicate entries. Standardizing data formats ensures a consistent and unified language for the AI model to understand. - Data Integration Strategies:
Once your data is clean, you’ll need to establish a seamless flow of information from various sources into the AI system. Consider employing data integration tools that can automate data extraction, transformation, and loading (ETL) processes. This ensures the AI model has real-time access to the most recent data for optimal performance.
Choosing the Right AI Solution
The retail AI landscape offers a diverse range of inventory forecasting solutions. Selecting the right one depends on your specific needs and resources.
- Cloud-based vs. On-premise Solutions: Cloud-based AI solutions offer a cost-effective and scalable option, particularly for smaller retailers. These solutions are typically subscription-based and require minimal upfront investment in hardware or software. On-premise solutions provide greater control over data security and customization, but require significant upfront costs and in-house technical expertise for maintenance.
- Industry Expertise and Scalability: Look for AI solutions specifically designed for the retail industry. These solutions will have pre-built models trained on relevant retail data, allowing for faster implementation and more accurate results. Consider scalability as your business grows. The chosen solution should be able to handle increasing data volumes and adapt to evolving business needs.
Change Management
Introducing AI into your inventory management processes can create a sense of apprehension among employees. Proactive change management strategies are crucial for ensuring a smooth transition and maximizing employee buy-in.
- Communication and Training:
Transparent communication about the goals and benefits of AI implementation is essential. Educate employees on how AI will complement their existing roles and empower them to make data-driven decisions. Provide comprehensive training on using the new AI system and interpreting its outputs. - Addressing Job Displacement Concerns: Reassure employees that AI is not intended to replace them, but rather to augment their capabilities. Focus on how AI will free up their time from tedious tasks, allowing them to focus on higher-value activities like customer service and strategic planning.
Conclusion
In conclusion, Retail AI offers a revolutionary approach to inventory management forecasting. By leveraging the power of machine learning and vast data analysis, AI can identify complex demand patterns, optimize stock levels, and predict potential disruptions. This translates into a multitude of benefits for retailers, including improved forecast accuracy, reduced stockouts and overstocking, optimized inventory levels, and ultimately, increased customer satisfaction and profitability.
While implementing Retail AI requires careful consideration of data quality, solution selection, and change management, the potential rewards are significant. Don’t be left behind in the retail revolution – explore the possibilities of AI inventory forecasting today. As a final word of advice, remember that AI is a powerful tool, but it’s not a magic bullet. Success hinges on a well-defined strategy, ongoing data management, and a commitment to continuous improvement. By taking these steps, you can unlock the true potential of AI and transform your inventory management for the better.
you may be interested in this blog here:-
Phonics Worksheets For Kindergarten PDF Fun Literacy Stations!
SOQL के साथ उपयोगकर्ता पहुँच का समस्या निवारण कैसे करें (शुरुआती के लिए अनुकूल)