Retail AI in Inventory Forecasting
Retail AI in Inventory Forecasting
- Historical Sales Data Analysis: Utilizes past sales trends to predict future inventory needs.
- Machine Learning Algorithms: Enhances accuracy of predictions by learning from ongoing sales patterns.
- Seasonal Trends Assessment: Adjusts forecasts based on seasonal buying behaviors.
- Real-time Data Processing: Incorporates current sales data for up-to-date forecasting.
- Supplier Performance Tracking: Monitors supplier reliability and adjusts inventory levels accordingly.
- Demand Forecasting Models: Applies statistical models to predict future product demand.
- Market Trend Analysis: Evaluates broader market trends to adjust inventory predictions.
The Necessity of Accurate Inventory Forecasting
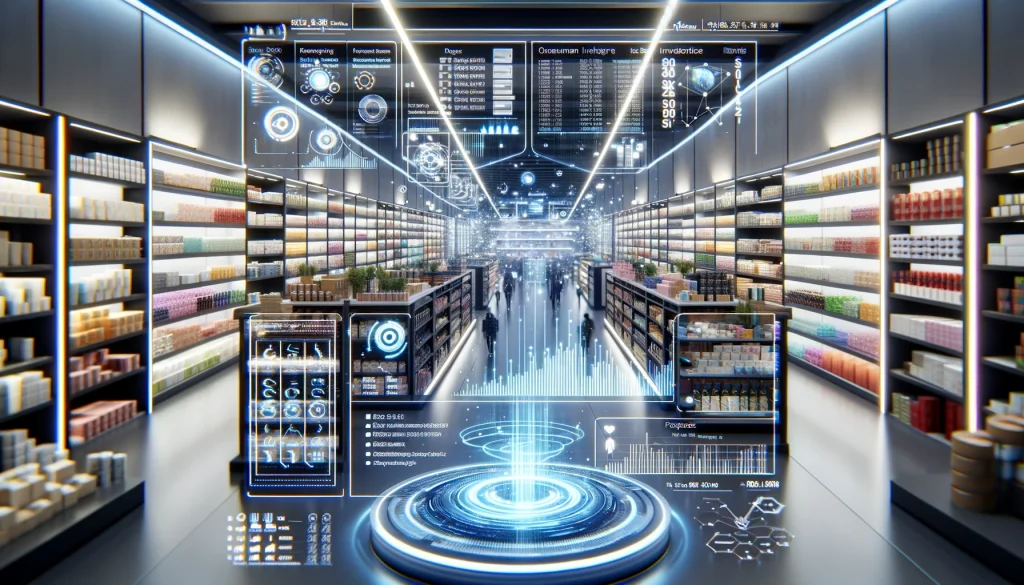
Inventory balance is a tightrope walk in the retail sector; excess and deficiency present challenges.
Accurate inventory forecasting is pivotal, ensuring this balance is maintained to foster retail success.
- Impacts of Mismanaged Inventory:
- Overstocking leads to increased holding costs, potential obsolescence, and wasted resources.
- Conversely, understocking can result in lost sales, diminished customer satisfaction, and weakened brand loyalty as consumers turn to competitors to meet their needs.
- Benefits of Optimization:
- Financial Advantages: Proper inventory forecasting reduces unnecessary expenditure and optimizes cash flow. By aligning stock levels with actual demand, retailers can maximize their profitability.
- Operational Efficiency: Streamlined inventory levels mean easier management, reduced storage costs, and a more agile response to market changes. This operational efficiency often translates into better customer experiences through consistent product availability.
AI’s Role in Inventory Forecasting
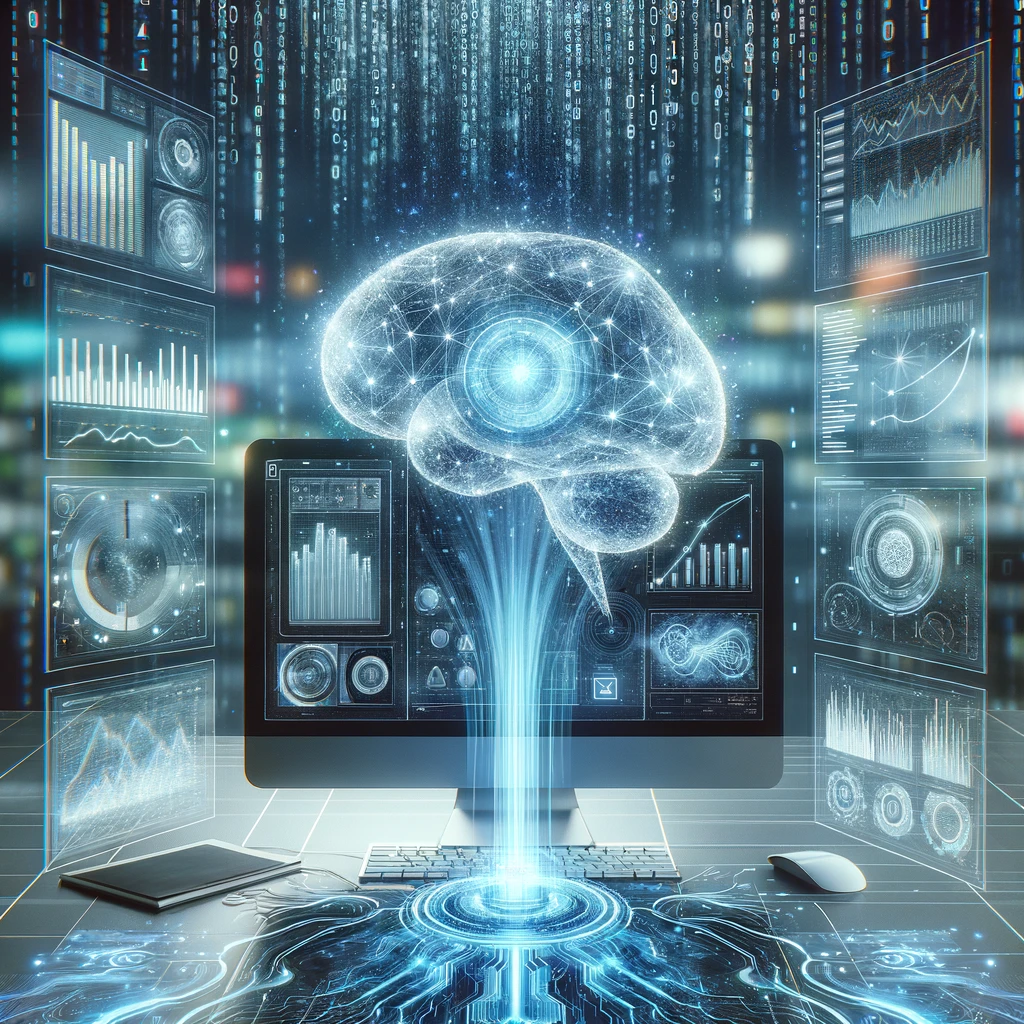
Artificial Intelligence (AI) is revolutionizing inventory forecasting by introducing levels of accuracy and efficiency previously unattainable with traditional methods.
- Predicting Future Requirements:
- AI leverages vast datasets encompassing sales trends, seasonal demands, and even broader market indicators to predict future inventory needs with remarkable precision. This foresight allows retailers to prepare for future demand without the risk of overstocking.
- AI Technologies at Work:
- Predictive Analytics: AI processes historical data to identify patterns and trends. AI can forecast future demand by analyzing past sales data, helping retailers make informed decisions about stock levels.
- Machine Learning (ML): ML algorithms go further by learning from data over time, continuously improving their predictions. These models adapt to changes in consumer behavior, seasonal shifts, and other variables, ensuring that inventory forecasts remain accurate and relevant.
By using AI for inventory forecasting, retailers can achieve a fine-tuned balance in their stock levels. Ensuring they have the right amount of product to meet demand without incurring unnecessary costs.
The transition to AI-driven forecasting represents a significant leap forward in retail inventory management, promising financial benefits and a boost in operational effectiveness and customer satisfaction.
Predictive Analytics in Detail
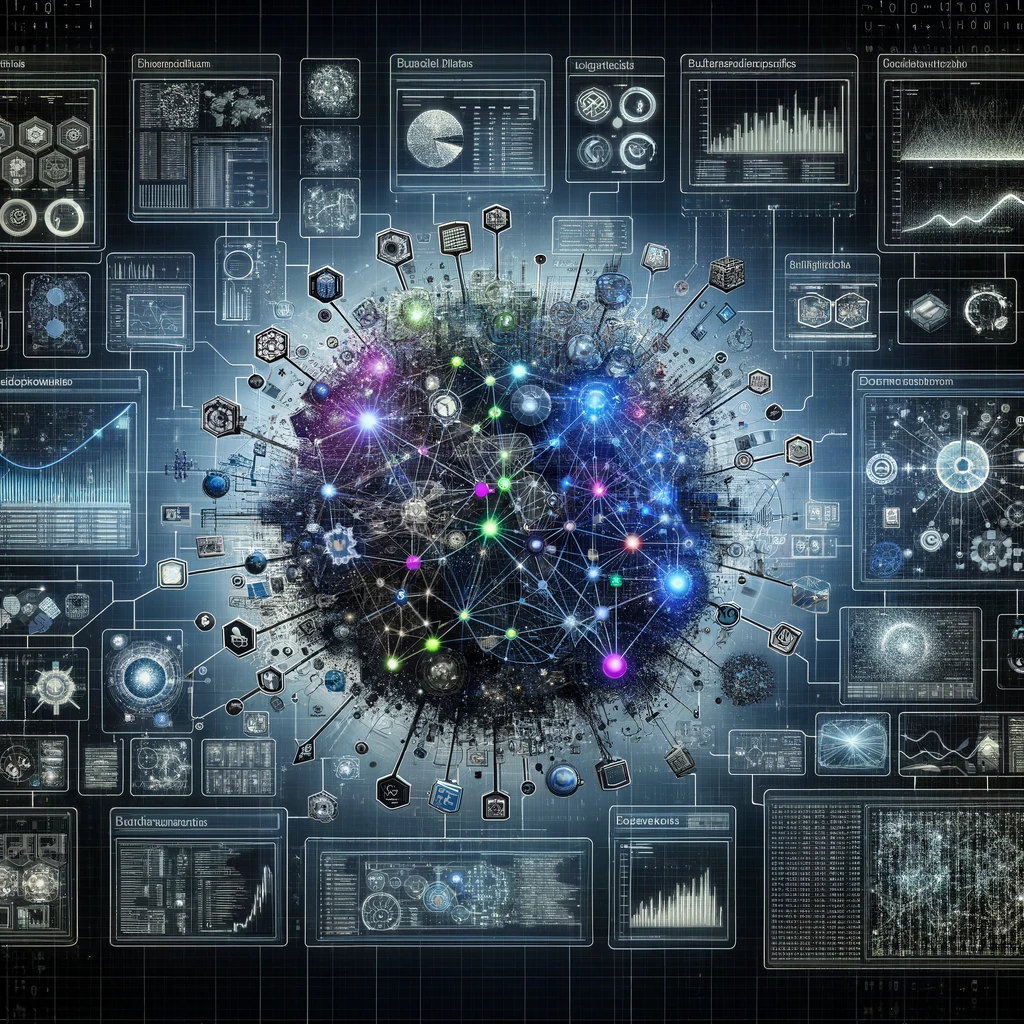
Predictive analytics has become a cornerstone in retail inventory management. It leverages historical sales data to create accurate forecasts of future demand.
This analytical approach combines various statistical techniques, including data mining and predictive modeling, to analyze past trends and predict future outcomes.
- Analyzing Historic Sales Data: By meticulously examining past sales figures, predictive analytics can identify patterns and trends likely to recur. This includes recognizing peak periods, seasonal fluctuations, and product life cycles.
- Case Studies:
- Fashion Retailer Optimization: A leading fashion retailer implemented predictive analytics to forecast demand for new clothing lines. By analyzing historical sales data, they adjusted their inventory orders accurately, resulting in a 20% reduction in unsold stock.
- Supermarket Stock Management: A supermarket chain uses predictive analytics to manage its perishable goods inventory. Predicting demand fluctuations based on historical sales, weather data, and holidays significantly reduces wastage and improves profitability.
Machine Learning for Dynamic Inventory Management
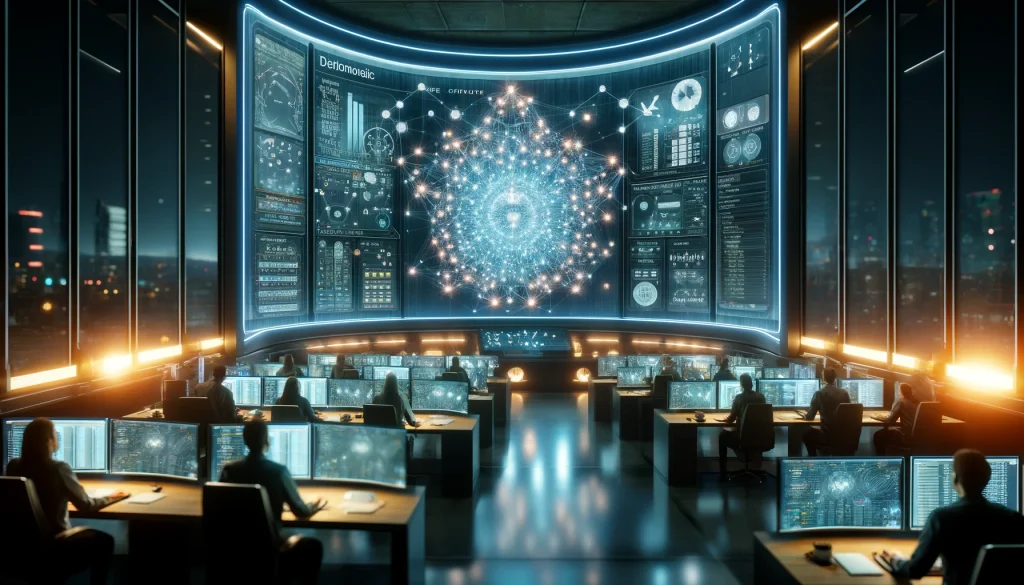
Machine learning (ML) takes inventory management further by analyzing past sales data and learning from it to make increasingly accurate predictions.
ML algorithms can adjust to changes in sales trends, seasonal demands, and unexpected market shifts, optimizing stock levels dynamically.
- Adapting to Sales Trends and Seasonal Demands: Machine learning algorithms continuously analyze sales data, adjusting their predictions for future demand based on real-time information. This allows retailers to maintain optimal stock levels, even during sudden market changes.
- Examples of ML in Retail Operations:
- Real-Time Stock Optimization: An electronics retailer implemented ML algorithms to adjust real-time inventory levels. This approach enabled them to respond quickly to changing consumer preferences, particularly for new tech gadgets, ensuring high-demand products were always in stock.
- Seasonal Demand Prediction: A home improvement store used ML to better understand seasonal trends affecting various product categories. The ML model predicted increased demand for gardening tools in spring and heating solutions in winter, allowing the retailer to adjust inventory accordingly and maximize sales.
By integrating predictive analytics and machine learning into their inventory management systems, retailers can forecast demand more accurately and ensure their stock levels are dynamically adjusted to meet consumer needs efficiently.
This strategic inventory management approach reduces costs associated with overstocking or stockouts and enhances the customer shopping experience.
Impact on Retail Operations
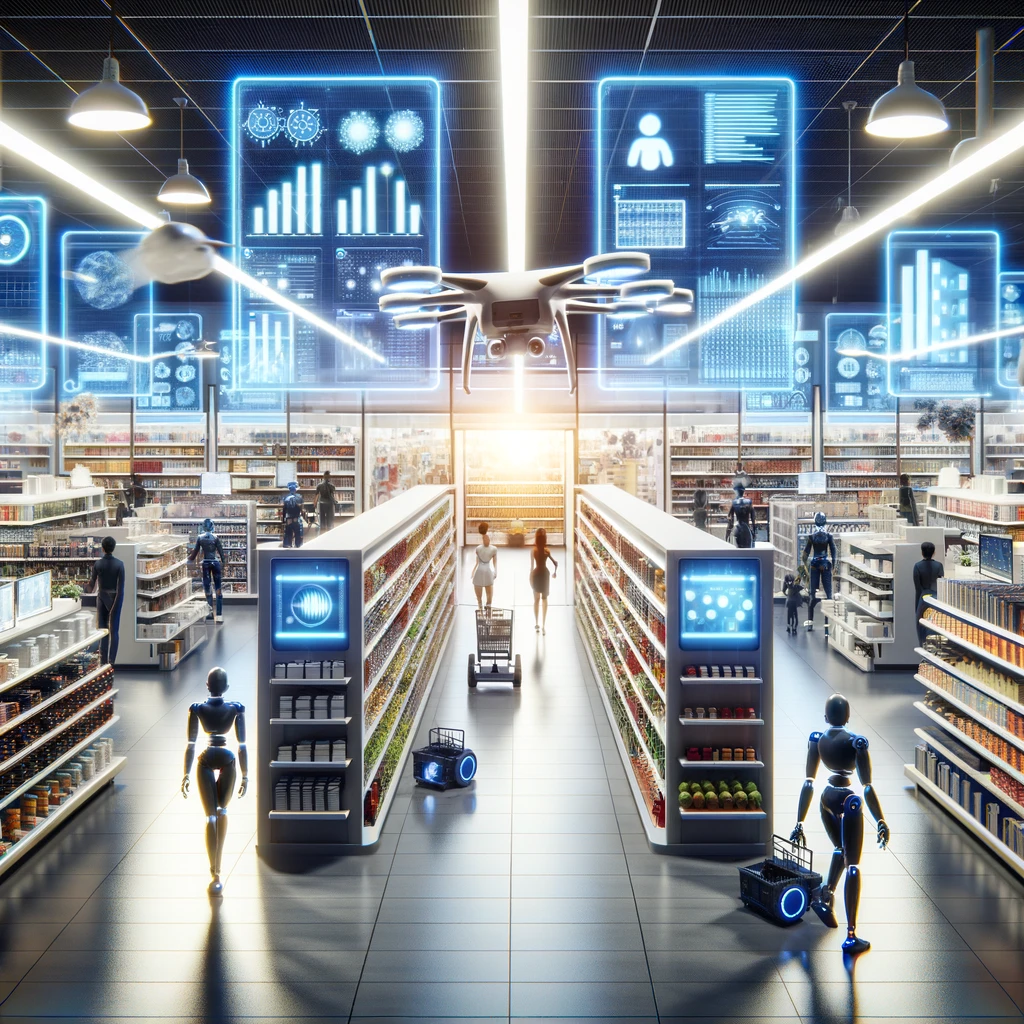
Integrating AI-driven inventory forecasting into retail operations heralds a new era of efficiency and accuracy.
By leveraging advanced algorithms to predict future demand, retailers can achieve stock optimization that significantly impacts their bottom line and customer satisfaction.
- Optimized Stock Levels: AI’s predictive capabilities ensure that retailers maintain the ideal stock balance—enough to meet demand without overstocking. This precision in inventory levels reduces holding costs and minimizes the risk of unsold inventory.
- Reduced Inventory Costs: Through accurate demand forecasting, AI helps retailers reduce excess inventory and associated costs. By investing only in stock that meets anticipated demand, retailers can allocate resources more effectively, enhancing profitability.
- Improved Customer Satisfaction: One of the most tangible benefits of AI-driven forecasting is the improvement in product availability. Customers are more likely to find what they need when they need it, boosting satisfaction and loyalty.
- Reduced Wastage: Especially relevant for perishable goods, AI’s ability to predict demand helps retailers order more accurately, thus reducing wastage and contributing to more sustainable business practices.
Implementing AI in Inventory Forecasting
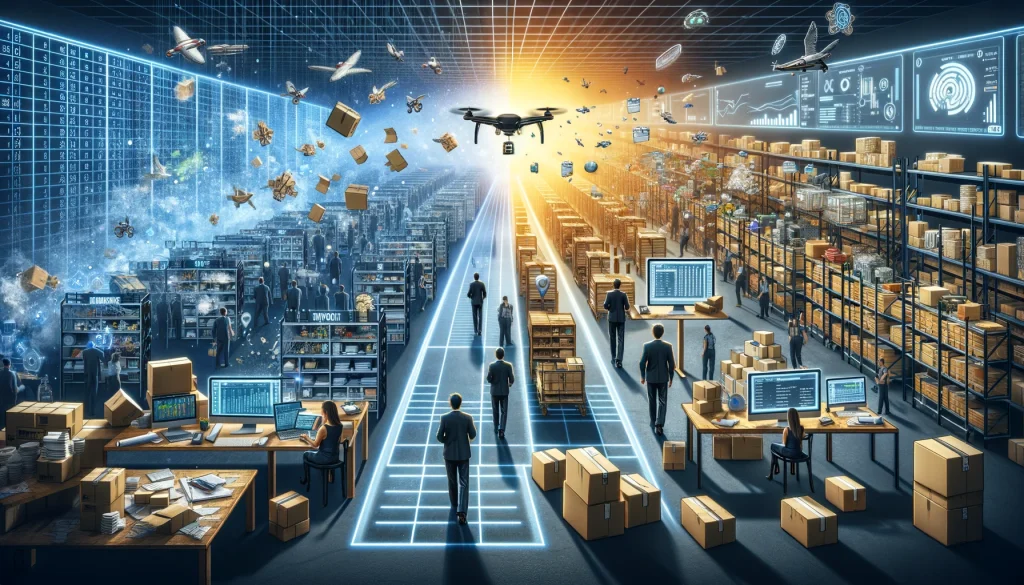
For retailers ready to embrace AI in inventory management, the journey from traditional methods to a technology-driven approach involves several key steps and considerations:
Steps for Integration:
- Assess Your Needs: Evaluate your current inventory management challenges and what you hope to achieve with AI. This will guide your choice of AI solution.
- Choose the Right AI Solution: Look for AI technologies specializing in inventory forecasting and offering features matching your retail sector and size.
- Data Preparation: Ensure your sales data, inventory records, and other relevant datasets are clean, accurate, and ready for analysis. AI’s effectiveness is directly tied to the quality of data it can learn from.
- System Integration: Work with IT specialists to integrate the AI solution with your inventory management system. Seamless integration ensures that AI-driven insights are effectively applied to inventory decisions.
- Training and Adoption: Train your team to use the new system and understand the insights it provides. Adoption across the organization is key to realizing the benefits of AI.
Considerations:
- Data Requirements: The accuracy of AI predictions depends on the volume and quality of data. Ensure you have access to comprehensive and high-quality sales and inventory data.
- System Compatibility: The AI solution should be compatible with your existing inventory management software and hardware infrastructure to avoid costly overhauls.
- Scalability: Choose an AI solution that can scale with your business, accommodating increased data volume and complexity as your operations grow.
Implementing AI in inventory forecasting transforms how retailers manage stock and offers a competitive edge in today’s fast-paced market.
AI-driven forecasting has become an indispensable tool in modern retail by optimizing inventory levels, reducing costs, and enhancing customer satisfaction.
Challenges and Considerations
Adopting AI for inventory forecasting presents several challenges and considerations that retailers must navigate to harness this technology’s full potential.
- Data Quality Concerns: AI’s predictive accuracy hinges on data quality fed into its algorithms. Inaccurate or incomplete data sets can skew forecasts, leading to suboptimal inventory decisions.
- Solution: Implement robust data collection and processing systems to ensure high-quality, comprehensive data for AI analysis.
- Privacy Concerns: The extensive data required for AI-driven forecasting, including customer purchase history, could raise privacy issues.
- Solution: Adhere strictly to data protection regulations, such as GDPR, and ensure transparent communication with customers about their data use.
Addressing these challenges involves a proactive approach to data management and privacy, ensuring the AI system has the foundation to operate effectively and ethically.
Future Trends in AI and Inventory Management
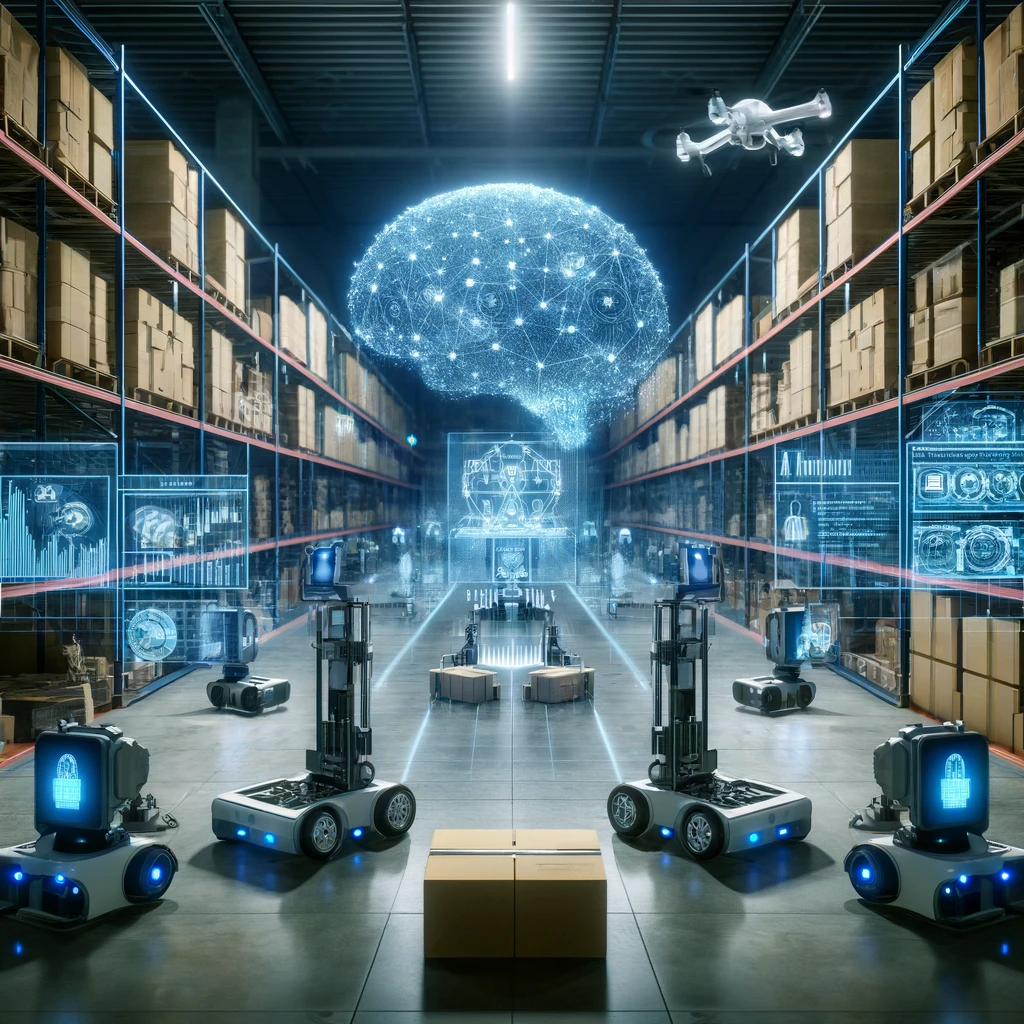
As AI technology evolves, its application in inventory management is set to deepen, offering new ways to refine and enhance retail operations.
- Predictive Accuracy Improvements: Continuous advancements in machine learning algorithms will further improve the predictive accuracy of AI systems, making inventory forecasts even more reliable.
- Integration with IoT: The Internet of Things (IoT) technology, combined with AI, could lead to more dynamic inventory systems. Sensors and smart devices in warehouses could provide real-time data to AI systems, enabling even more nuanced inventory adjustments.
- Automated Restocking: Future AI systems may directly control the restocking process, automatically placing orders with suppliers when inventory levels dip below-predetermined thresholds, streamlining the supply chain.
- Customized Consumer Experiences: AI could use inventory data with customer preference insights to personalize shopping experiences, suggesting products more accurately and improving stock levels for high-demand items.
Emerging technologies and innovations promise to make AI-driven inventory forecasting more effective and integrate inventory management into a broader strategy for retail optimization.
The future of AI-powered retail operations will likely be marked by greater efficiency, reduced costs, and enhanced customer satisfaction.
FAQs
How does Historical Sales Data Analysis improve inventory forecasting?
Analyzing past sales trends helps retailers predict future inventory needs by identifying patterns and fluctuations in product demand over time.
What role do Machine Learning Algorithms play in forecasting?
Machine Learning Algorithms improve the accuracy of inventory predictions by continuously learning from and adapting to new sales patterns and data.
Why is Seasonal Trends Assessment important for inventory management?
Assessing seasonal trends allows retailers to adjust their inventory forecasts based on expected changes in buying behavior during different times of the year, such as holidays or seasonal shifts.
How does Real-time Data Processing impact inventory forecasting?
Real-time data processing enables retailers to incorporate the latest sales data into their forecasts, making predictions more current and responsive to market changes.
What is the purpose of Supplier Performance Tracking in inventory forecasting?
Tracking supplier performance helps retailers adjust their inventory levels based on suppliers’ reliability and lead times, ensuring stock availability matches demand.
Can you explain Demand Forecasting Models?
Demand Forecasting Models use statistical methods to analyze sales data and predict future product demand, helping retailers make informed inventory decisions.
How does Market Trend Analysis assist in adjusting inventory predictions?
Market Trend Analysis involves evaluating broader market and industry trends to refine inventory forecasts, considering factors like consumer behavior changes and economic conditions.
Is inventory forecasting accurate for all types of products?
While inventory forecasting can significantly improve accuracy, its effectiveness varies by product type and market conditions, with some items being more unpredictable than others.
How often should inventory forecasts be updated?
Inventory forecasts should be regularly updated to reflect new sales data, market trends, and changes in consumer behavior. Some retailers do so daily or weekly.
What impact do promotional events have on inventory forecasting?
Promotional events can significantly impact demand, requiring adjustments to inventory forecasts to account for anticipated increases in sales during promotions.
Can inventory forecasting reduce stockouts and overstock?
Effective inventory forecasting can minimize stockouts and overstock by aligning inventory levels more closely with actual demand.
How do external factors influence inventory forecasting?
External factors such as economic shifts, weather events, and changes in consumer preferences can influence demand patterns, necessitating adjustments to inventory forecasts.
What technologies are used in inventory forecasting besides machine learning?
Technologies used in inventory forecasting include data analytics platforms, cloud computing for data storage and processing, and ERP systems for integrating sales and inventory data.
How can small retailers implement inventory forecasting?
Small retailers can implement inventory forecasting by using simpler demand forecasting tools and software, focusing on analyzing sales data and key demand drivers relevant to their market.
What future developments can we expect in inventory forecasting?
Future developments may include more advanced AI and machine learning models, greater integration of external data sources, and more collaborative forecasting involving suppliers and partners.