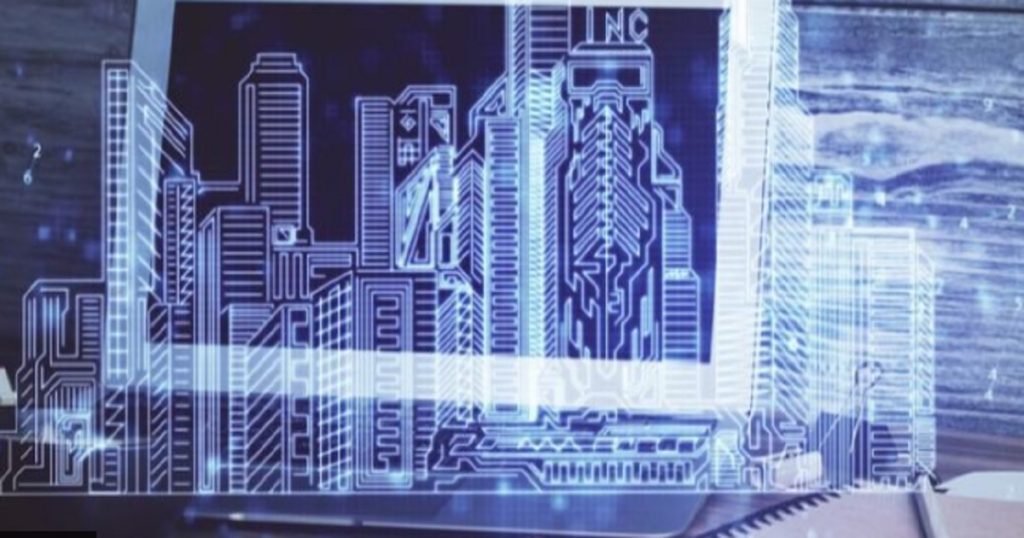
Many big data projects I have been asked to review were in critical condition and the root cause always was that the team followed the rulebook on how to build a data lake to the letter.
One of these big data rules is to transform the data at query time instead of preparing the key performance indicators (KPI) upfront and allow only those to be analyzed.
All too often the rule is executed by dumping all data as-is into the data lake. CSV files, database exports, spreadsheets, text documents,… it is chaos. As a result the data is physically present but essentially inaccessible.
Late binding
A data warehouse is supposed to be the single point of truth. Everybody can query the “Revenue” and everybody has the same definition of what “Revenue” contains and what it doesn’t. While this is a good idea for many measures, the definition of others is not always as clear.
In the weblog analysis the KPI “reading duration” is defined as “time between accessing one page and within 10 minutes another”. Why 10 minutes? What would be the impact on the calculated duration if using 9 minutes instead? While it is definitely a good idea to provide a KPI with the official definition “reading duration” for correlations, the data scientist will want to do more.
Hence the requirement to make the raw data (and consequently big data) accessible. But does that also mean in the original source format, meaning a 1:1 copy of the webserver logs? What will happen if that data gets converted from the text format into a compressed binary format easier to read? Would that violate one of the data lake rules if 100 percent of the information is present but in a different format?
New deal
Because storing the data in databases was the only option in the past, many data sources could not be added to the data warehouse or only in a pre-aggregated fashion. Today, I would use the data lake as data warehouse staging area on steroids.
All data are added in a binary compressed columnar table format. Parquet format* is the defacto standard. This makes it easier for the data scientist as well as the data warehouse to consume the data. The data warehouse still contains the aggregated data, using the official definition of the KPI.
Data science
The reason the data warehouse plays a significant role in this context is because of its ease of use and speed. Most of the users only care about the predefined KPIs. Their typical analysis is to create correlations between the data. And that is the sweet spot for databases like SAP Hana – smaller volumes, fast aggregations, fast search, many joins.
A data lake is the exact opposite. It can store unlimited amounts of data cheaply, can parallelize processing on a single table efficiently and provides complex processing way beyond SQL queries.
The combination of the two, data lake and data warehouse, is the winning proposition.